Introduction
Table of Contents
In the expansive world of artificial intelligence, the prowess of language models is undeniable as they forge new paths in understanding and replicating human speech. In-context learning stands at the forefront, redefining the mastery of language for these digital intellects. A breakthrough technique, known as iterative forward tuning boosts in-context learning in language models, has emerged, signaling a leap towards refining AI efficacy. This piece examines the core of in-context learning and demystifies iterative forward tuning, highlighting its divergence from traditional methods and its pivotal role in the progress of language models.
Understanding In-Context Learning
Definition and Importance
In-context learning enables models to absorb and react based on the input provided during their use, bypassing the need for further training sessions. Its value lies in granting models the ability to tailor their responses dynamically, making the interaction more fluid and saving invaluable time and energy.
Challenges in In-Context Learning
However, in-context learning is not without hurdles, primarily in ensuring consistent and reliable outcomes across varying contexts. Another hurdle is the requirement for a diverse set of examples which is crucial for the model’s effective learning, yet this can limit its application.
Examples of In-Context Learning in Action
Examples abound, such as sophisticated chatbots that tailor their responses to the flow of conversation or email sorting systems that categorize messages by analyzing their content, demonstrating in-context learning’s practical significance.
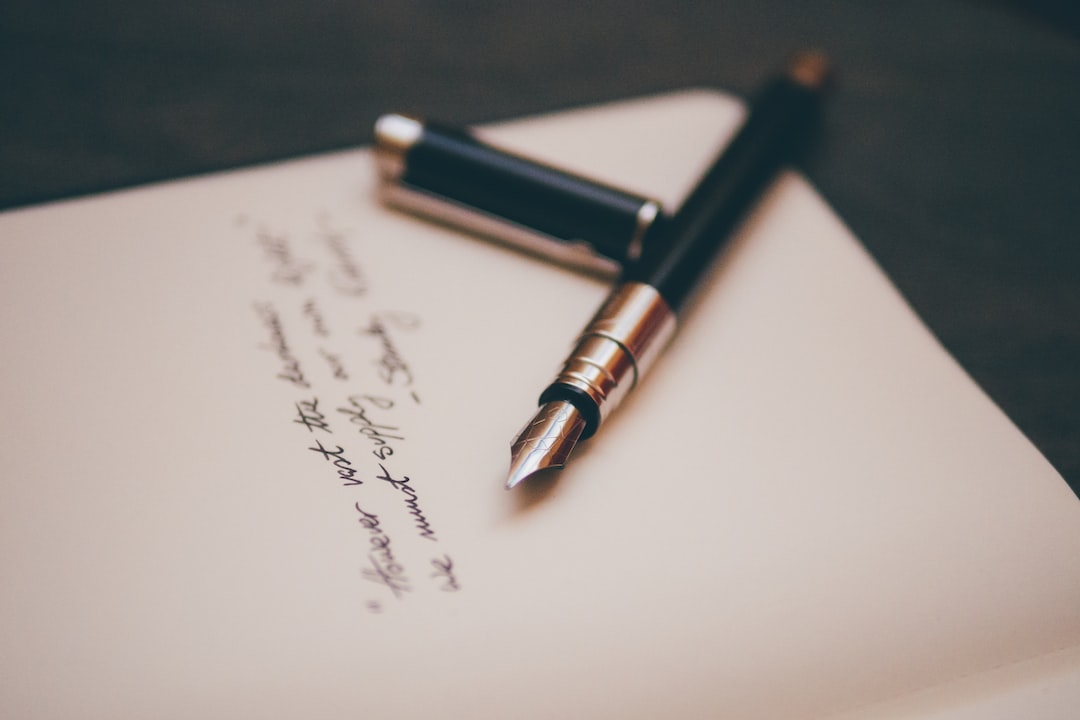
The Basics of Iterative Forward Tuning
What is Iterative Forward Tuning?
Iterative forward tuning boosts in-context learning in language models, offering a novel edge. This method, akin to how humans learn gradually, involves incremental refinements to a model, enhancing its language processing abilities through continuous updates.
The Process of Iterative Forward Tuning
IFT unfolds in stages, beginning with fine-tuning a model using a curated dataset, followed by evaluation and a repeated cycle of refinements based on the output from the latest model iteration, fostering perpetual improvement.
Comparing IFT to Traditional Fine-Tuning
Compared to traditional fine-tuning, which typically ends after one training round post-initial pre-training, IFT’s iterative cycle continuously integrates fresh data, ensuring the model stays relevant and performs effectively.
Enhancing Language Models with IFT
Improvements in Language Understanding
Iterative forward tuning boosts in-context learning, significantly enriching a model’s grasp of language nuances, allowing more precise interpretations of complex phrases and idiomatic expressions.
Increased Efficiency in Model Training
Another boon of IFT lies in its enhanced training efficiency. Constant improvements nullify the need for starting from scratch, leading to a more cost-effective and manageable development process for intricate language models.
Case Studies of Success
Evidence of IFT’s prowess is seen in various success stories, from models fine-tuned for deciphering legal terms to those adept at medical diagnostics or providing culturally aware translations.
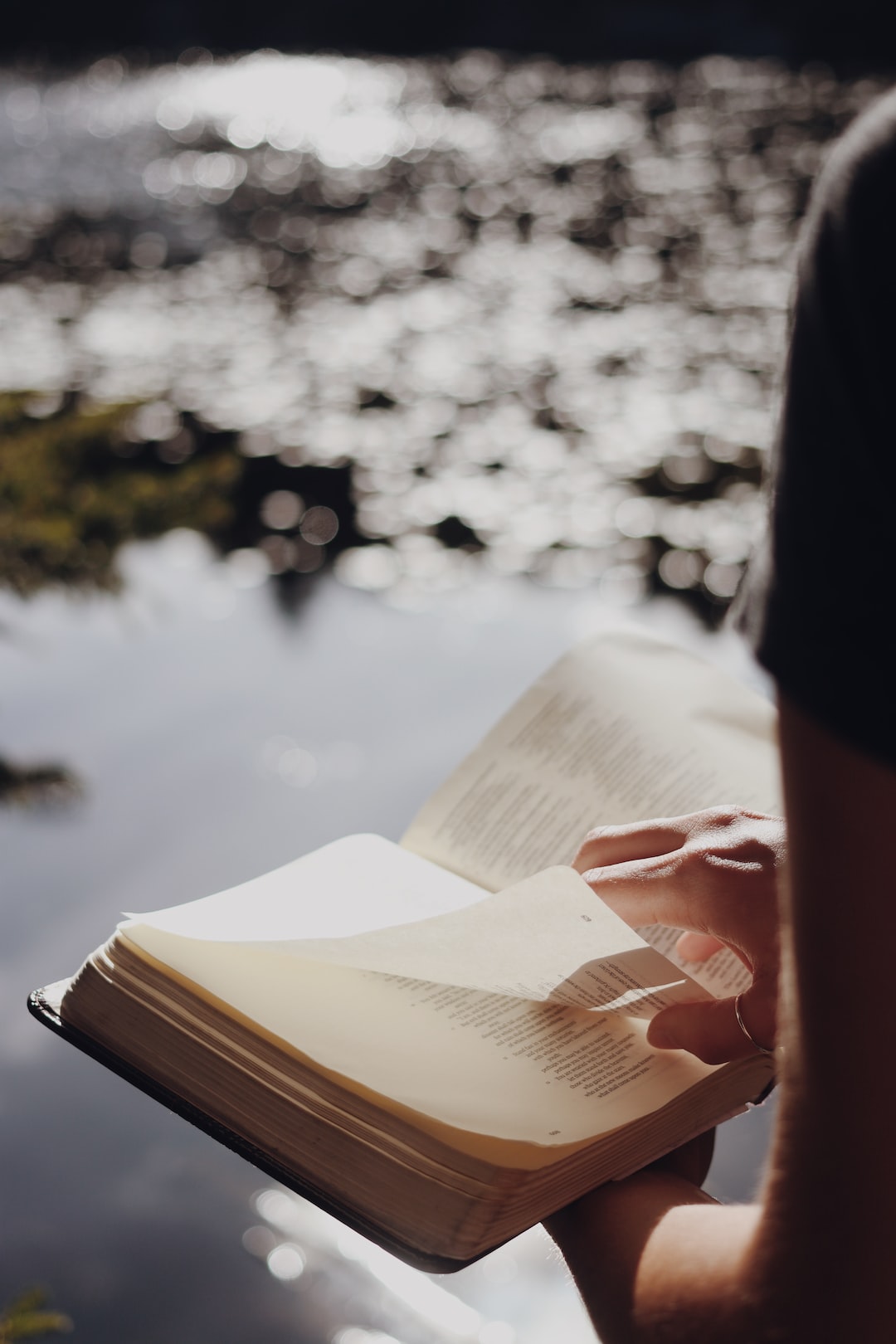
Key Benefits of IFT for Language Models
Adaptability to New Contexts
Iterative forward tuning boosts language models’ adaptability, allowing them to integrate fresh data with ease and adjust to evolving linguistic trends.
Better Handling of Ambiguity and Nuance
Dealing with the inherent vagueness and subtleties of language is made more manageable through IFT, as ongoing exposure to varied contexts hones the models’ sensitivity to linguistic intricacies.
Streamlining Model Deployment
IFT also streamlines the deployment process, as models can continue to improve even while in use, reducing interruptions usually linked with updates and upkeep. In similar efforts to enhance language acquisition, the effective use of intelligent flashcards can play a pivotal role in reinforcing language skills.
Practical Applications of IFT in Language Processing
Real-World Use Cases
From enhancing customer service bots to refining AI-generated content, the application of iterative forward tuning boosts in-context learning across numerous industries, making real-world interactions smoother and more intuitive.
IFT in Multilingual Models
Multilingual models too have been enriched by IFT, enhancing their performance across various languages and thereby expanding their usability on a global scale.
Improving Machine Translation Quality
Machine translations have also been elevated thanks to IFT, aiding in producing translations that are not only accurate but also culturally coherent, signalling progress in the realm of computational linguistics.
Challenges and Limitations of IFT
Computational Resource Requirements
The iterative process integral to IFT needs significant computational power and memory, which could hinder organizations lacking the necessary tech.
Potential Overfitting Issues
Overfitting poses a risk with IFT, as repeated tuning on the same datasets can lead to models that are overly specialized and less effective with novel inputs.
Scalability Considerations
Lastly, scaling IFT to accommodate growing data and user demands remains a challenge that developers must navigate carefully.
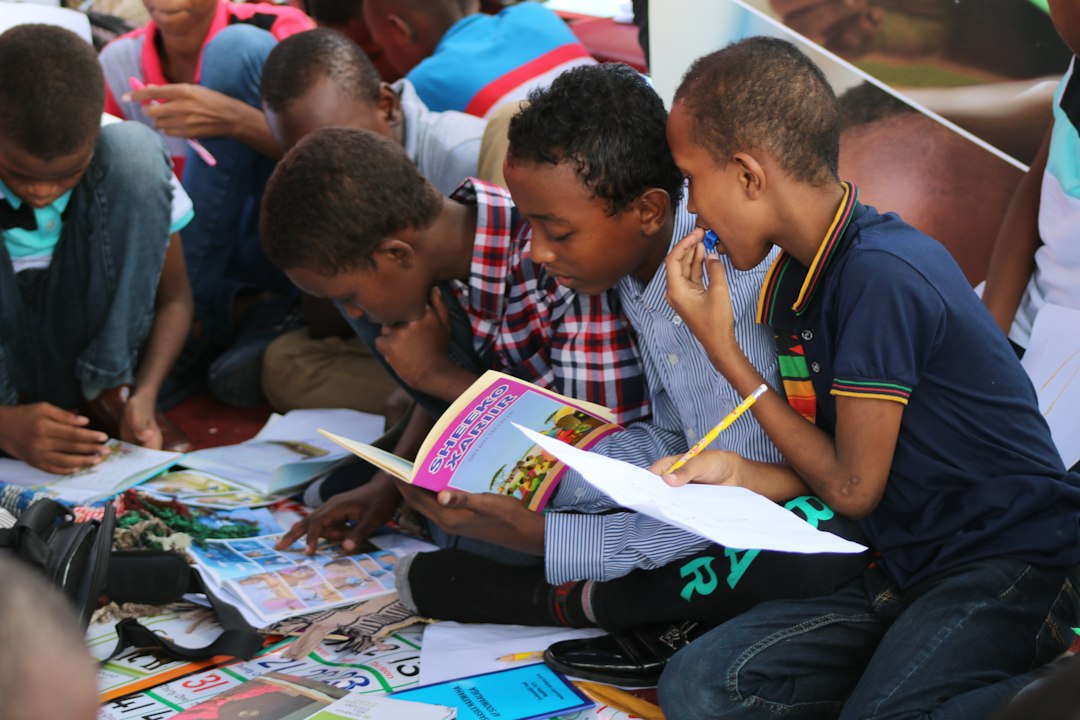
Future of IFT and In-Context Learning
Research Directions and Potential Breakthroughs
Research endeavors aim to refine IFT further, with the promise of models capable of learning from an even broader spectrum of contexts with less oversight.
Integration with Emerging Technologies
Merging IFT with novel technologies like quantum computing could enhance its efficiency exponentially, heralding a new epoch for language models.
Predictions for the Evolution of Language Models
Speculations suggest language models will progress in sophistication, with iterative forward tuning playing a key role, potentially achieving human-like interactive capabilities.
Conclusion
Iterative forward tuning boosts in-context learning in language models, marking a significant stride in AI linguistics. It embodies the fusion of innovation and pragmatism, advancing the limits of AI in language understanding. With continued exploration and innovation, the future holds untold potential for the synergy between language and machine learning, propelling us towards discoveries that are currently beyond our imagination.
FAQs: Understanding Iterative Forward Tuning in Language Models
- What is iterative forward tuning and how does it enhance in-context learning in language models?
- Iterative forward tuning is a method that improves in-context learning by incrementally refining a language model, allowing it to process language with greater nuance and efficiency. By continually integrating new data, this technique keeps the model relevant and effective over time.
- How does iterative forward tuning differ from traditional model fine-tuning?
- Unlike traditional fine-tuning, which generally concludes after a single round of post-initial training, iterative forward tuning involves a cyclical process of ongoing refinement. This ensures that the model adapts to new data and contexts, improving its performance consistently.
- What are some practical applications of iterative forward tuning in the real world?
- Practical applications of iterative forward tuning range from enhancing conversational AI like chatbots to improving machine translation quality, ensuring translations are not only accurate but culturally coherent. It’s employed across industries to make interactions with technology more seamless and intuitive.
- Are there any challenges associated with implementing iterative forward tuning?
- Yes, iterative forward tuning requires substantial computational resources and can be prone to overfitting if not managed properly. It also faces scalability challenges, as adapting to growing data sets and user demand can be complex.
- What future advancements might we see with iterative forward tuning in language models?
- Future advancements may include even more efficient iterative forward tuning methods, possibly with reduced supervisory needs. It’s also expected to benefit from merging with emerging technologies like quantum computing, driving language models towards a level of sophistication akin to human-like interactions.